![]() |
|
||||
BiographyBalázs Bámer was born in 1976, and received his MSc in 2001 at the Technical University of Budapest, where he studied computer science. After several years in industrial software development and various jobs in academia, he joined Institute for Microelectronics in January 2023 as a doctoral candidate in the Christian Doppler Laboratory for Multi-Scale Process Modeling of Semiconductors Devices and Sensors. His research focus is on studying dopant implantation and activation. In particular, he is working on a model for dopant activation in SiC, based on ion implantation conditions, such as temperature. |
Cluster-Based Physical Model for Dopant Activation in Silicon Carbide
Technology Computer-Aided Design (TCAD) tools are essential for simulating and designing semiconductor manufacturing processes. They can substitute time-consuming and expensive experiments by accurately simulating various processing steps like ion implantation, annealing, etching, oxidation, etc. Simulations are especially important for assessing newer semiconductor materials like silicon carbide (SiC), for which there is a lack of experimental knowledge in science and industry.
Today, mainly ion implantation is applied to dope semiconductors because it allows for precise control of the doped region geometry and the doping concentration. Its main drawback is its induced damage to the semiconductor crystal structure. This damage weakens the semiconductor's electrical properties by making some dopant atoms electrically inactive. Inactive dopant atoms combine in smaller or bigger clusters instead of residing in lattice positions. This change of properties depends on the ion flux, the ion energy, and the implantation temperature. Subsequent processing steps, like annealing, can be used to repair the crystal structure and activate the dopant atoms.
Depending on the damage caused by ion implantation, SiC may require temperatures as high as 2000°C during annealing. Physical simulations may reveal that a lower temperature or shorter annealing time might be sufficient when the annealing effect of subsequent processing steps like thermal oxidation are considered. We aim to develop a simulation model capable of predicting the added annealing effect of different processing steps. The current empirical models cannot simulate these added effects since they are analytical models only considering the implantation conditions, such as the implant dose and temperature. They are also unable to model the effects of sequential processes, such as multiple annealing steps at different temperatures.
We are building a physical model to describe the behavior of the various species in the semiconductor during annealing. These are the dopants on lattice points, point defects, dopant-defect pairs, and clusters of different sizes. We define the possible reactions between them, including the reaction kinetics, and use a set of ordinary differential equations (ODEs) to model the time evolution of the concentration of the different species during annealing. The same ODEs are also suitable for modeling the combined effects of reactions and diffusion in a commercial TCAD framework. An optimizer is implemented to accompany this model to elucidate the post-implant conditions of the SiC film, including the various cluster concentrations. These concentrations are not measurable and can only be calculated through time-intensive atomistic simulations, which we will apply to verify and calibrate our model.
Our work consists of a model generator, which takes a compact model description and produces the ODEs. The second part is a global optimizer used to find the initial concentrations and missing parameters by tuning them to make the model fit experimental data for the activation rate under various conditions. Other missing parameters are obtained from ab initio density functional theory (DFT) and molecular dynamics (MD) simulations.
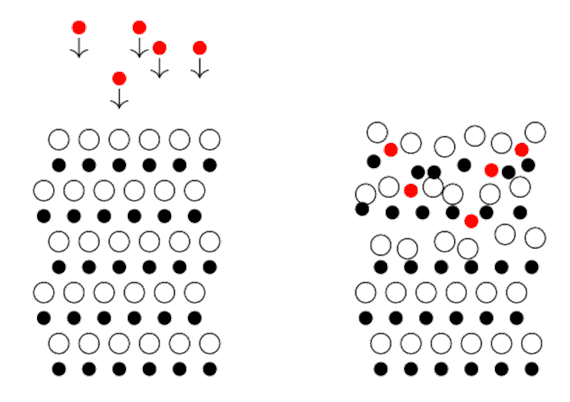
Fig. 1: Ion implantation into silicon carbide and the resulting lattice damage.
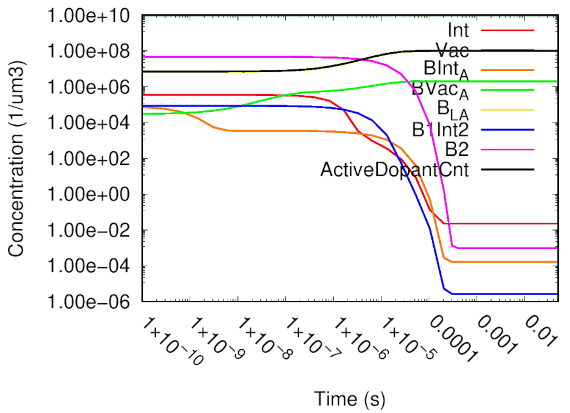
Fig. 2: Time evolution of concentrations of different species during annealing simulation.