![]() |
|
||||
BiographyJohannes Ender was born in 1988 in Hohenems, Austria. In 2013 he finished his Master’s studies at the University of Applied Sciences in Vorarlberg. After working in industry for three years he pursued the Master’s studies of Computational Science at the University of Vienna. In November 2018 he joined the Institute for Microelectronics where he started his PhD studies researching the simulation of non-volatile magnetic memory devices. |
Improving the Energy Efficiency of SOT-Assisted STT-MRAM with Reinforcement Learning
Nonvolatile memory is a promising option to replace CMOS-based memory devices, with the most common types of magnetoresistive RAM (MRAM) cells being spin-transfer torque (STT-)MRAM and spin-orbit torque (SOT-)MRAM. Combining these two mechanisms has led to improvements in MRAM performance. Based on previous research which has demonstrated the use of reinforcement learning (RL) for SOT-MRAM switching, we have expanded our framework to encompass different MRAM types and reward functions.
By allowing the agent to control the STT and SOT pulses individually, and by providing it with state information of the memory cell containing magnetization and magnetic field values, the agent was trained to reverse the magnetization. The results of an agent trained with a reward function which simply penalizes the deviation from the target z-value (-1) can be seen in Fig. 1. It successfully reverses the magnetization, while leaving the STT pulse turned on, which is unacceptable with respect to power consumption, but also for practical use. Fig. 2 provides a visual representation of an improved reward function, which encourages fast magnetization reversal and little power consumption. It is always negative unless the magnetization in the memory cell is completely reversed. The reward function can be divided into three domains, with varying levels of power penalty. The highest power consumption results in the most negative reward, while the negative reward, when both pulses are turned off, is a result of the deviation of the current magnetization value from the target value. An agent which is trained using this reward function applies pulses to reverse the magnetization, as shown in Fig. 3. Magnetization reversal can still be achieved, although over a longer time period, and the agent can slow down the rate at which the negative reward is accumulated, as evidenced from the kinks in the accumulated reward. This indicates that the agent is attempting to increase energy efficiency, which is also reflected in the significant reduction in write energy as compared to the results shown in Fig. 3.
By appropriately selecting the reward function, an RL agent can be motivated to learn pulse sequences for switching SOT-assisted STT memory cells in a more energy-efficient manner. Therefore, with the help of machine learning-aided approaches, like the one presented here, energy consumption of future devices can be significantly reduced.
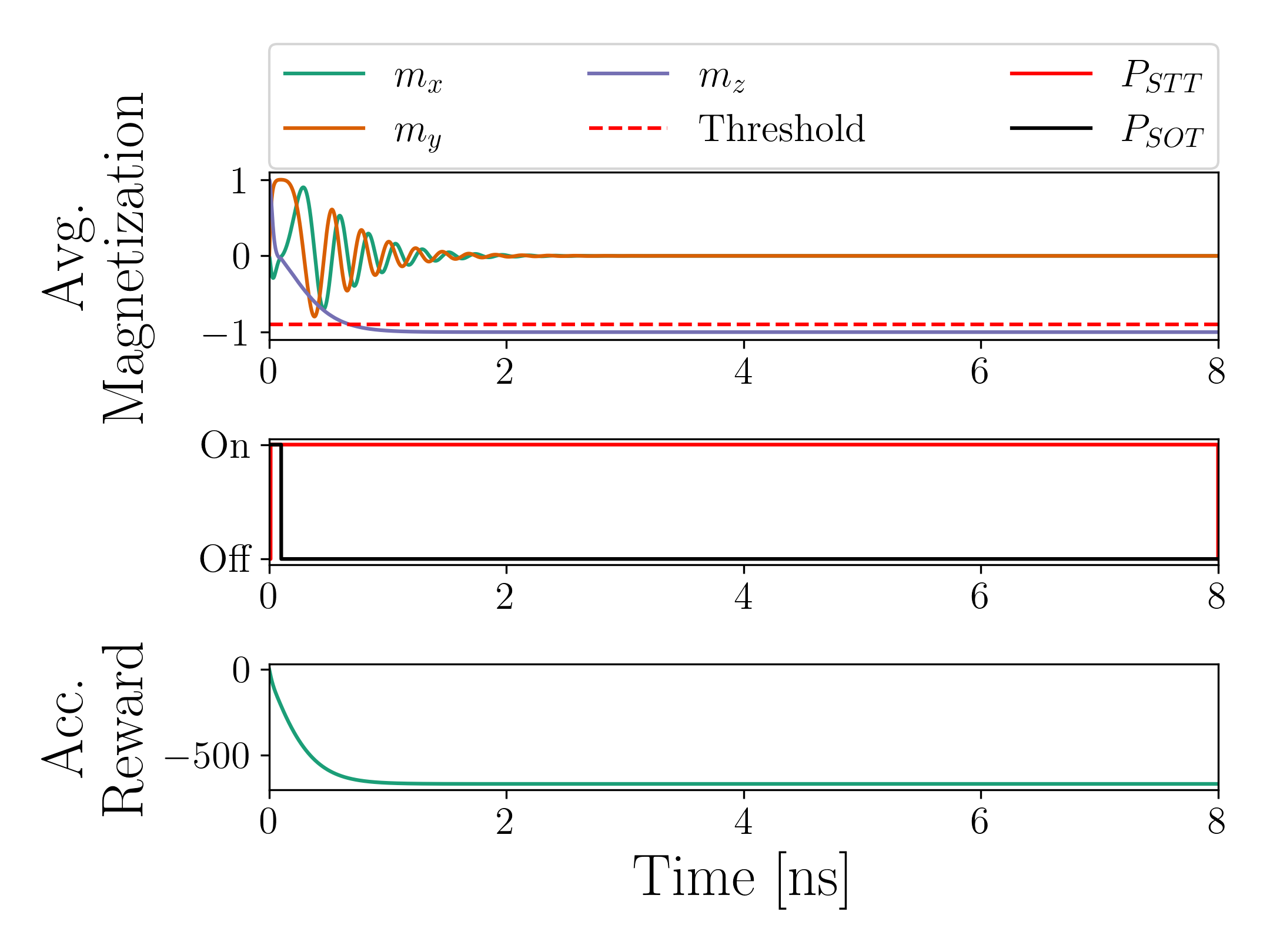
Fig. 1: Model trained without penalizing power consumption.
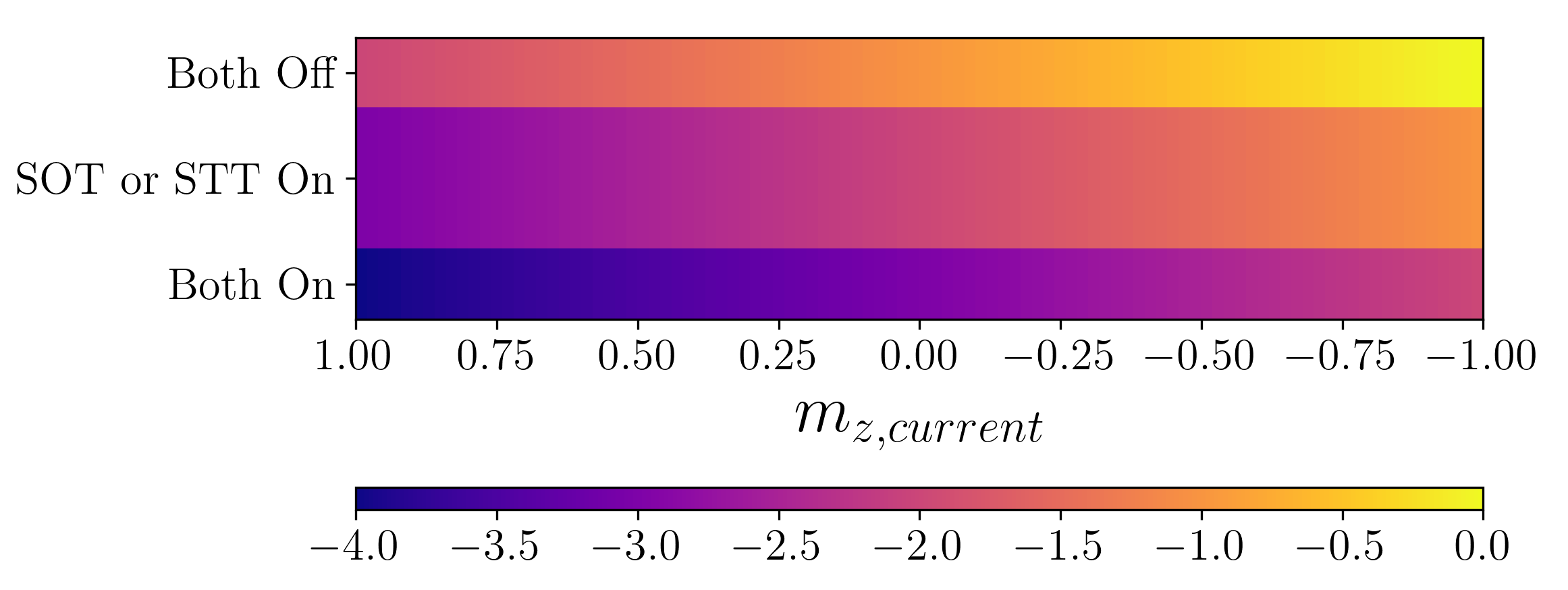
Fig. 2: Reward function with a power consumption penalty. Color coding represents the reward returned after each iteration.
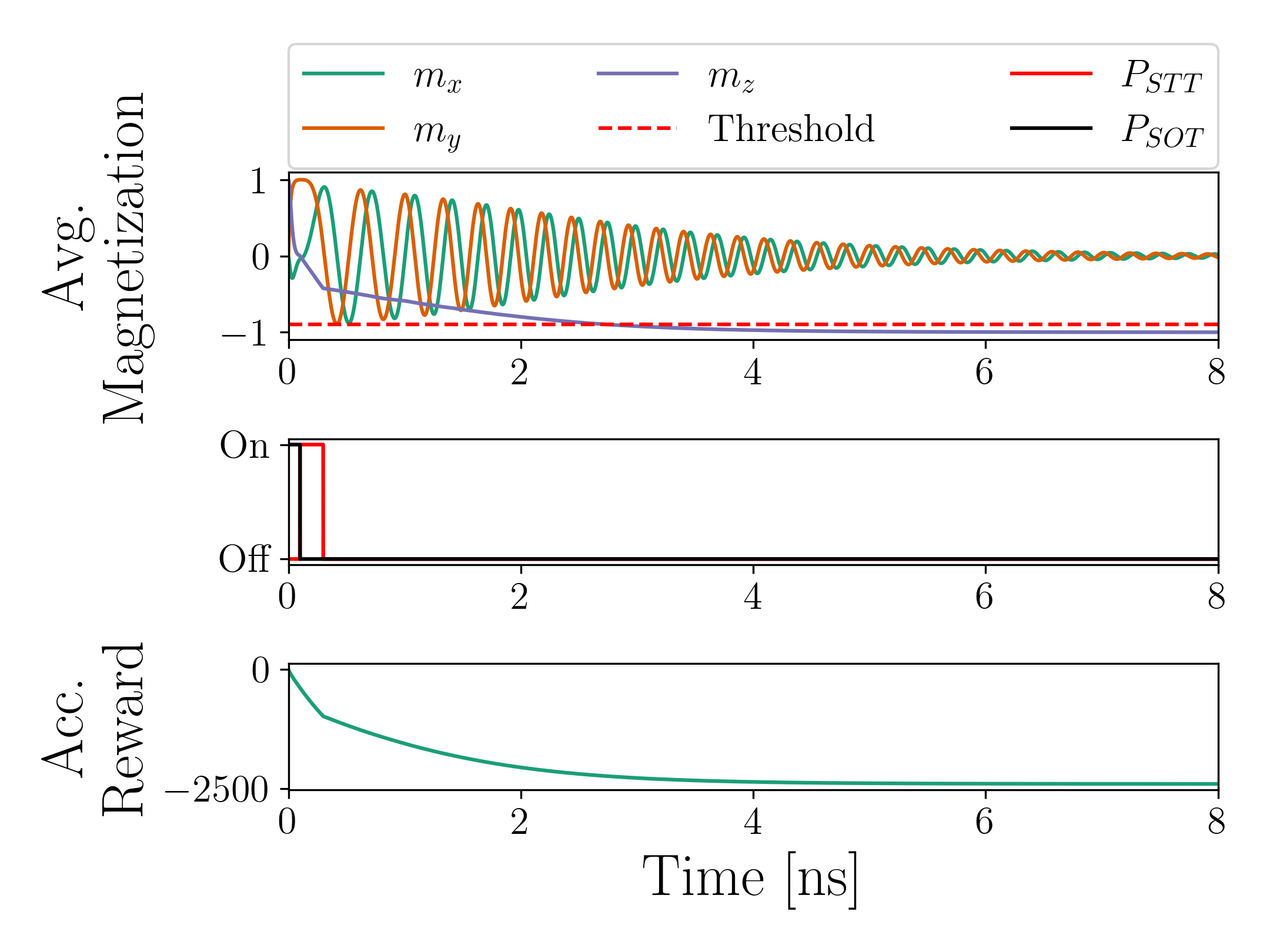
Fig. 3: Model trained with a reward function which penalizes power consumption.